Intel Igpu Or Cpu Folding – Folding Performance Review!
Explore how Intel iGPU or CPU folding compares to competitors like NVIDIA and AMD in performance for Folding@home and distributed computing tasks.
Folding On Intel Arc Cards: How Intel’s New Igpu Competes With Cpu Folding!
Overview of Intel Arc Cards and Folding Performance!
This analysis compares the capabilities of Intel’s integrated graphics processing units (iGPUs) with those of traditional CPUs in folding applications.
Intel Arc iGPUs: Performance and Architecture!
The B580 model features 20 Xe cores and a clock speed of 2670 MHz, while the A580 has 24 Xe cores but a lower clock speed of 1700 MHz. These advancements contribute to a performance increase that can be significant compared to older integrated solutions.
- Key Specifications:
- Architecture: Intel Xe2
- Memory: Up to 12 GB GDDR6
- Transistor Count: 19.6 billion for B580
- Performance: Up to 45% higher in benchmarks compared to competitors like Nvidia.
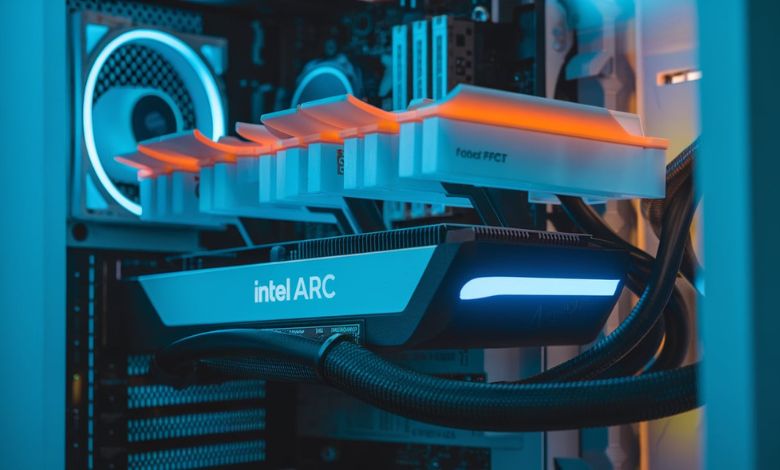
Comparative Performance: iGPU vs. CPU Folding!
- Integrated Graphics:
- Recent benchmarks show that Intel’s iGPUs can handle F@H tasks but are not optimized for high-performance folding due to their limited compute capabilities.
- Users report varied success with iGPUs like the HD Graphics series, which can perform basic folding tasks but yield lower scores compared to dedicated GPUs.
- CPU Folding:
- Traditional CPUs, particularly those from Intel’s Core series, are generally more capable of handling F@H due to their architecture designed for versatile computing tasks.
- CPU performance in folding scenarios often surpasses that of integrated graphics due to higher core counts and better support for the necessary computational frameworks.
Also Read: Frustum Clipping On Cpu – Speed Up Your Rendering!
CPU vs GPU Work Units: A Deep Dive into Folding at Home Performance!
Here are some insightful resources on the topic of “CPU vs GPU Work Units: A Deep Dive into Folding at Home Performance”:
CPU vs GPU work units
This forum thread discusses the differences in how CPUs and GPUs handle work units in Folding at Home, and the relative performance between the two.
Is it worth folding on a CPU?
A Reddit discussion exploring whether it’s worth using CPUs for Folding at Home, including comparisons to GPU performance.
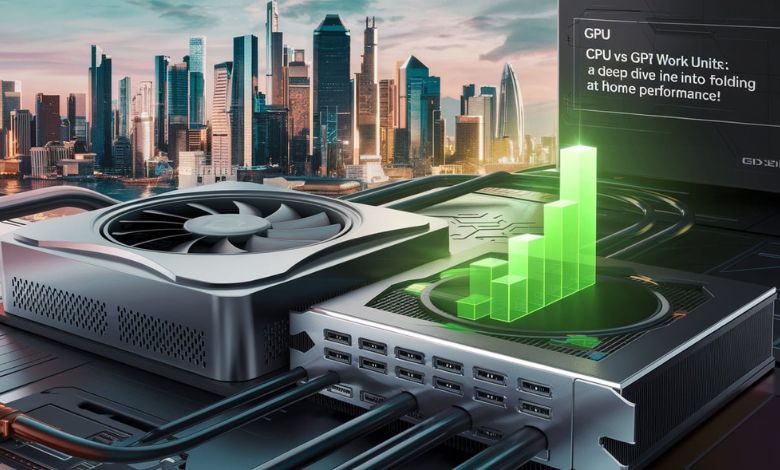
Folding At Home Cpu Vs Gpu
An article highlighting how GPUs outperform CPUs in Folding at Home due to their parallel processing abilities.
Cpu Vs Gpu: How They Work And When To Use Them
A comprehensive guide comparing the architecture and use cases of CPUs and GPUs, which provides context on why GPUs excel in parallel computing tasks like Folding at Home.
Also Read: b75 motherboard what generation cpu – Complete Guide!
Maximizing Folding Performance: CPU + GPU Folding Setup Best Practices!
Here are best practices to optimize your setup effectively.
Use the Latest Folding Client
- Upgrade to v7 Client: The v7 client is designed to manage multiple hardware components efficiently, allowing you to run both CPU and GPU folding simultaneously. It automatically configures slots for your hardware, making it user-friendly and effective for maximizing performance.
Optimize Slot Configuration
- Configure CPU and GPU Slots: After installing the v7 client, pause the SMP (Symmetric Multi-Processing) slot and switch to Advanced or Expert mode in FAHControl. Adjust the CPU allocation in the SMP settings:
- Change the CPUs field from -1 (all cores) to a number that leaves one thread free for each GPU. For example, if you have two GPUs, set it to 6 on an 8-core CPU. This prevents interference from GPU tasks, allowing more efficient CPU folding.
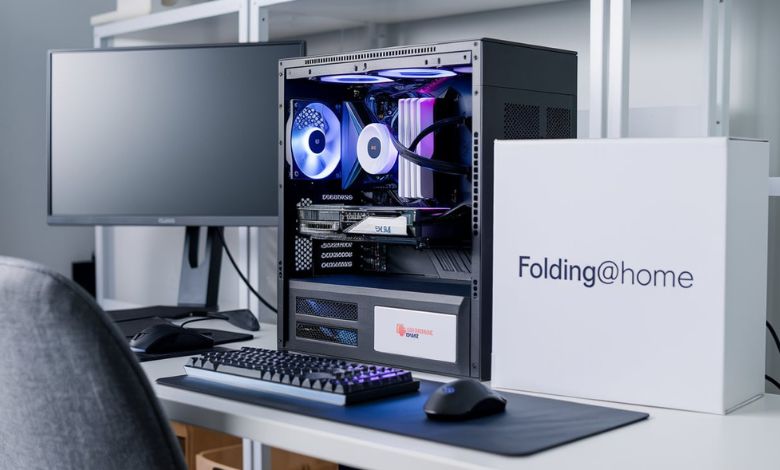
Manage Resource Allocation
- Balance CPU and GPU Workloads: Each CPU core can typically support two GPUs. If you have multiple GPUs, ensure that your CPU has enough threads available to handle their workloads without bottlenecking performance.
Monitor Temperature and Power Usage
- Adjust Power and Temperature Limits: To maintain stability during intensive folding tasks, consider lowering the power and temperature limits of your GPUs. This can prevent overheating and potential throttling during long folding sessions.
Avoid Conflicting Tasks
- Pause Folding During Gaming or Heavy Usage: GPU folding does not mix well with other GPU-intensive tasks like gaming due to a lack of scheduling software for GPUs. If you plan to engage in other demanding activities, pause the GPU folding slots to avoid performance degradation.
Test Stability Regularly
- Run Stability Tests: Ensure that your system is stable under folding loads by using stress testing tools like StressCPU v2, which mimics the workload of F@H. Regularly check for stability as overclocking settings may not always translate well into folding performance.
Keep Software Updated
- Regular Updates: Ensure that both your operating system and the Folding@Home client are kept up-to-date. Updates often include performance improvements and bug fixes that can enhance overall efficiency.
Folding With Intel Cpus: Are They Competitive With Modern Gpus?
Here’s a detailed analysis based on recent benchmarks and user experiences.
Performance Comparison: Cpus Vs. Gpus
- Raw Processing Power: Modern GPUs are specifically designed for parallel processing and excel at handling the types of calculations required for F@H. For example, a typical Nvidia GTX 1650 can achieve around 262,389 points per day (PPD), while an Intel i7-9750H CPU may only reach approximately 42,337 PPD under similar conditions.
- Efficiency and Points per Day (PPD): The PPD for CPUs varies significantly based on the model and architecture. For instance, the 13th Gen Intel Core i7-13700K has been reported to achieve competitive PPD values, but still lags behind high-end GPUs like the RTX 3080 or AMD’s RX 6800 XT, which can exceed several million PPD when fully utilized.
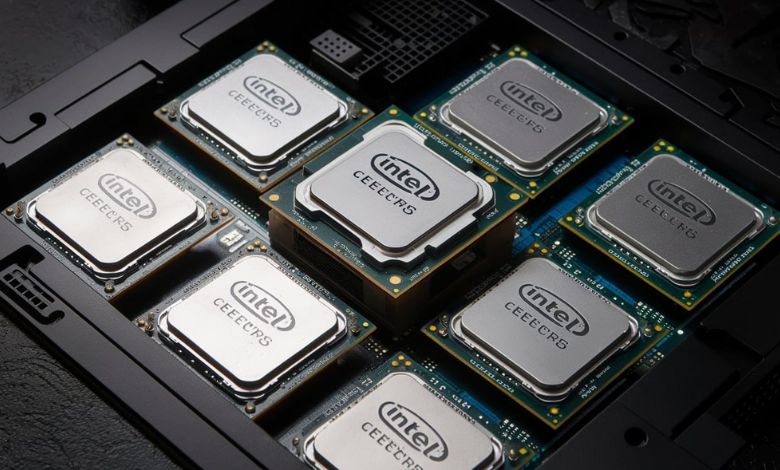
Architectural Considerations
- Big.LITTLE Architecture: Intel’s recent CPU designs (12th and 13th generations) utilize a hybrid architecture combining Performance cores (P-cores) and Efficiency cores (E-cores). This design can complicate resource allocation in F@H, as some users have reported issues with thread management leading to reduced performance when both CPU and GPU are active.
- Single-threaded vs. Multi-threaded Performance: F@H benefits from multi-threading capabilities; however, many workloads within F@H can still be single-threaded. This means that while modern Intel CPUs can handle multiple threads efficiently.
Practical User Experiences
- Users have noted that while Intel CPUs can contribute to folding efforts, they often do not match the efficiency of dedicated GPUs. For example, when running intensive tasks on a high-end GPU like the RX 6800 XT alongside an Intel CPU, users have reported significant drops in PPD when attempting to utilize both resources heavily.
- The consensus among folders is that while Intel CPUs can be part of a folding setup, especially for those who do not have access to powerful GPUs, they are generally not competitive with modern GPUs in terms of overall folding efficiency and output.
Also Read: Can Cpu Cause Crashes – How to Fix Overheating and Errors!
Intel’s Market Position in GPU Folding: Competing Against AMD and NVIDIA!
Here’s a detailed analysis of Intel’s current market standing and its competitiveness in GPU folding.
Current Market Position
- Market Share Decline: As of Q2 2024, Intel’s share of the discrete GPU market has plummeted to 0%, a stark decline from a minimal 2% share just a year prior. This shift highlights Intel’s inability to gain traction in a market dominated by Nvidia, which commands 88%, and AMD, holding 12% of the market.
- Sales Dynamics: The overall sales of discrete graphics cards have increased significantly, with shipments reaching 9.5 million units in Q2 2024, marking a 9% increase from the previous quarter. However, Intel has not benefited from this growth, as all gains have been captured by Nvidia and AMD.
- Product Launch Challenges: Despite launching its Arc series GPUs, Intel has struggled to follow up with new products that could compete effectively against Nvidia’s latest offerings. The anticipated Battlemage architecture has been delayed, leaving Intel without a competitive edge in the rapidly evolving GPU landscape.
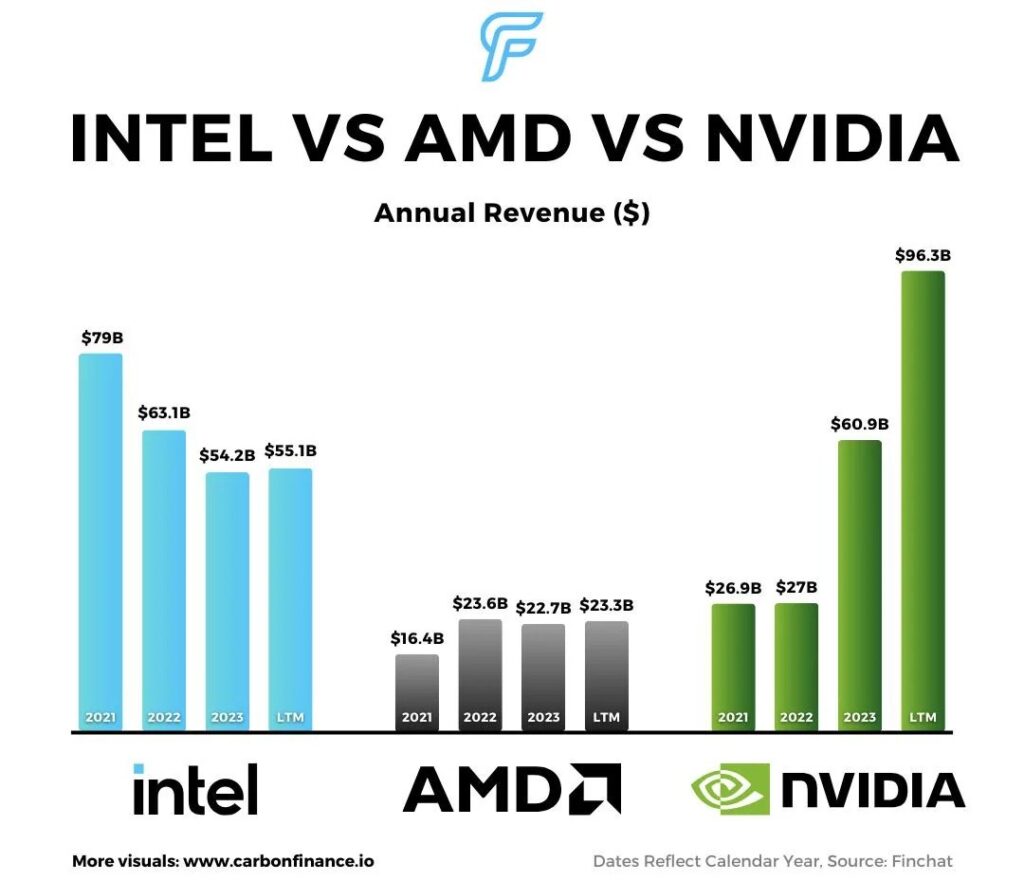
Competitiveness in GPU Folding
- Folding Performance: In terms of performance for Folding@Home (F@H), modern GPUs from Nvidia and AMD are vastly superior to Intel’s offerings. GPUs are designed for parallel processing, which is crucial for the computations involved in folding tasks.
- User Experience and Adoption: Users have reported that while Intel CPUs can contribute to folding efforts, they are generally not competitive with dedicated GPUs. The lack of robust support for F@H on Intel’s GPUs further hampers their adoption among folding enthusiasts who prioritize efficiency and output.
- Future Outlook: Analysts suggest that for Intel to reclaim any meaningful market share in the discrete GPU sector, it would require significant advancements in both product development and software compatibility. The company is focusing on refining its product lineup and addressing existing issues.
Intel’s Decline In The Discrete Gpu Market: What It Means For Folding Performance!
Here’s a breakdown of the situation and its potential impacts:
Intel’s Entry Into The Discrete Gpu Market
However, despite significant investments and initial excitement, Intel has faced several challenges, including:
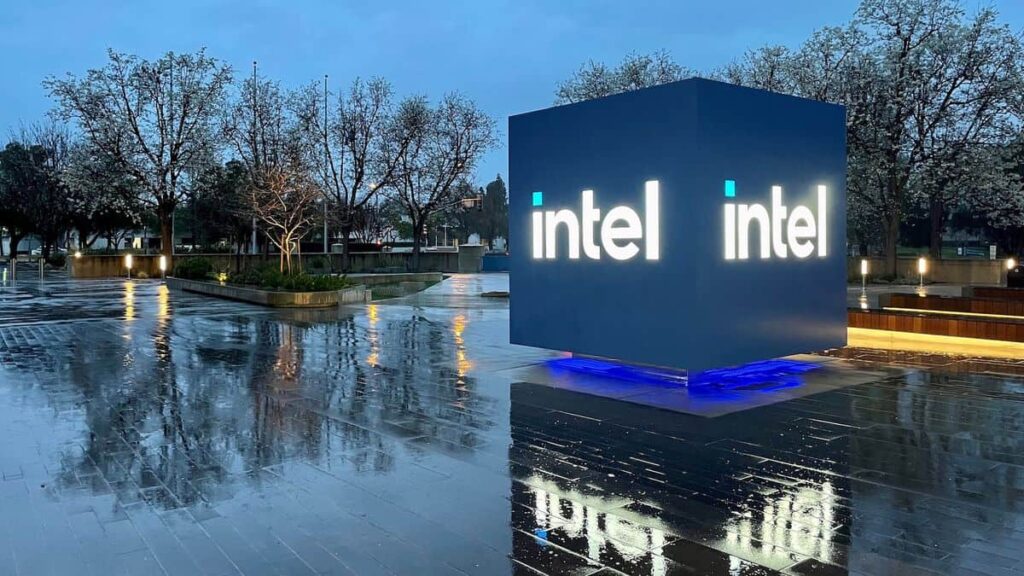
- Performance and Driver Issues: Intel’s discrete GPUs struggled to match the raw performance of NVIDIA and AMD’s offerings, particularly in high-demand areas like gaming and professional workstations. Many users reported stability and driver issues that hampered the overall experience.
- Market Positioning: While Intel had the advantage of its strong CPU market presence, it faced stiff competition in the discrete GPU segment, where NVIDIA and AMD had established strong footholds with highly optimized hardware and software solutions.
- Supply Chain Constraints: Intel faced challenges in scaling production, which affected availability and performance consistency. This impacted consumer confidence and limited the market penetration of its GPUs.
Also Read: Can You Play With Just a Cpu – Exploring the Power of Just a CPU!
Impact on Folding Performance
Intel’s decline in the discrete GPU market can have several implications:
Lack of Optimized Hardware for Folding:
- Folding Performance on Intel GPUs: The Intel Arc GPUs, despite being marketed as high-performance options, haven’t been optimized for the specific needs of folding applications. While NVIDIA and AMD GPUs have a longer history of providing robust support for computational science projects like Folding@home, Intel’s Arc series struggled to deliver the level of performance needed for these tasks.
- Limited CUDA/ROCm Support: NVIDIA’s CUDA technology and AMD’s ROCm platform are integral to achieving high efficiency in distributed computing projects like Folding@home. Intel’s Arc GPUs don’t yet offer native support for either, limiting their integration with the Folding@home software, which relies on these platforms for performance optimizations.
Fewer Contributions From Intel-Based Systems:
- Underutilized Market: Given the struggles of Intel’s discrete GPU line, many potential contributors who might have purchased Intel-based GPUs for Folding@home likely turned to more reliable, high-performance alternatives from NVIDIA or AMD.
- Declining Appeal of Intel Arc for Folding: With Intel’s GPUs not being a primary choice for high-performance computing applications like Folding, researchers might have to continue relying on the more established ecosystems of NVIDIA and AMD.
Future Prospects:
- Intel’s Strategy for Growth: While Intel’s first attempt at the discrete GPU market has faltered, the company is not likely to abandon the field altogether. Future iterations of Intel GPUs may come with improved performance and better driver support, which could make them more appealing for computational tasks like Folding@home.
- Collaborations and Software Support: For Intel to recover its position in the discrete GPU market and improve folding performance, it would need to establish strong partnerships with scientific computing platforms like Folding@home and contribute to enhancing software compatibility, similar to what NVIDIA has done with CUDA.
Also Read: Does Streaming Heat Up Cpu – Tips to Keep Your System Cool!
FAQS: About intel igpu or cpu folding
Does Folding@home use CPU or GPU?
Folding@home can use both CPU and GPU, but GPUs are much faster. GPUs handle parallel tasks better, making them ideal for protein folding simulations. CPUs can be used, but they are slower compared to GPUs.
Why did Intel’s GPU fail?
Intel’s GPUs struggled with performance and driver issues. They couldn’t match the speed and reliability of AMD and NVIDIA. Many users found Intel’s GPUs less optimized for high-demand tasks like gaming or folding.
Is it better to use CPU or GPU for Blender?
GPU is generally better for Blender, especially for rendering. It speeds up tasks like 3D modeling and animation due to its parallel processing. However, CPUs can still be used for some tasks, but they are slower than GPUs.
What is the most efficient GPU for Folding@home?
NVIDIA GPUs, particularly the RTX 30-series and 40-series, are considered the most efficient for Folding@home. They offer excellent performance and are well-optimized for folding simulations. AMD GPUs are also good but generally less efficient for this task.
Conclusion
Intel iGPU or CPU folding is an option, but it lags behind more powerful solutions like NVIDIA and AMD GPUs. While Intel’s integrated GPUs (iGPUs) and CPUs can contribute to Folding@home, they don’t offer the same level of efficiency or speed needed for large-scale protein simulations. For the best performance in folding tasks, dedicated GPUs from NVIDIA and AMD remain the top choice, offering superior processing power and optimized software support.